The College of Tourism and Service Management has always adhered to the motto " Dedication to public interests, acquisition of all-round capability, and aspiration for progress with each passing day" and has been keeping a close eye on the forefront of technological development, devoting itself to promoting the construction of the theoretical, disciplinary and discourse systems of China's distinctive tourism industry. This has injected a strong impetus into the high-quality development of the tourism industry under the new development pattern. The research team led by Associate Professor Bi Jianwu, a selected member of the "One Hundred Young Disciplinary Leaders Program of Nankai University", is dedicated to promoting research on deep analysis and intelligent decision-making of big data. The team uses advanced big data analysis and artificial intelligence technology to conduct in-depth analysis and mining of tourism big data and develops effective prediction and decision-making support models to promote the intelligent, digital and information-based development of the tourism industry.
Dr. Bi Jianwu graduated from Northeastern University and is currently an associate professor and doctoral supervisor at the College of Tourism and Service Management, Nankai University. He was selected for "One Hundred Young Disciplinary Leaders Program of Nankai University" and serves as a director of the Intelligent Decision and Game Theory Committee of China's Preferred Law and Economic Mathematics Research Association, and the Management and Decision Science Committee of China's Management Modernization Research Association. Dr. Bi is the leader of two national-level scientific research programs and multiple provincial-level scientific research programs, and has been a core member of nearly 10 programs supported by the National Natural Science Foundation, National Social Science Foundation, and the Humanities and Social Science Fund of the Ministry of Education. Associate Professor Bi Jianwu has published more than 30 high-quality research achievements in top journals of tourism disciplines and related disciplines such as Tourism Management, Annals of Tourism Research, Journal of Travel Research, International Journal of Contemporary Hospitality Management, International Journal of Production Research, Information Sciences, Information Fusion, and Tourism Economics in the past five years. His research has attracted wide attention from scholars abroad and has been cited nearly 1500 times on Google Scholar. Two of his papers have been selected for the ESI hot papers list, and three of his papers have been selected for the ESI highly cited papers list.
Dr. Bi Jianwu has led a team focusing on research in the field of big data and decision analysis. They have conducted extensive and in-depth explorations in the areas of tourism big data deep analysis and intelligent decision-making, and have achieved fruitful research results. Dr. Bi has made significant contributions to promoting the progress of big data intelligent mining in Nankai University's tourism management discipline. Their specific research work includes:
1. Proposing a method for improving the design of tourism and service products based on online reviews.
A large amount of online review information for various products can be seen on e-commerce websites, OTA, and other platforms with the rapid development of information technology. These online reviews contain valuable information that can support the improvement and design of tourism and service products. How to analyze massive online review information to improve the design of tourism and service products is a research topic with important theoretical significance and practical application value. Therefore, the team has systematically and relatively early investigate product improvement design methods based on online reviews and proposed a series of analysis methods and technologies. Compared with the traditional method of using survey questionnaires, the analysis method based on online review data can save a lot of time and economic costs and provide real-time, scientific recommendations for the improvement of tourism and service products. Over the past five years, Dr. Bi Jianwu has published seven SCI/SSCI-indexed papers as the first author or corresponding author related to this topic, which has been cited more than 400 times and two of which have been selected as ESI highly cited papers. Representative research achievements have been published in top international journals such as Tourism Management, Annals of Tourism Research, and International Journal of Production Research, and have been directly cited and positively evaluated by Professor K Sudhir, former editor-in-chief of the top marketing journal Marketing Science from the Yale University, Professor Alan Wang, senior editor of the famous international journal Electronic Commerce Research and Applications from the Virginia Tech, Professor Harrison Kim, area editor of famous international journal IISE Transactions Design and Manufacturing from University of Illinois at Urbana-Champaign, Professor Heesup Han, editorial board members of internationally renowned journals such as Tourism Management and Tourism Review from Sejong University in Korea, Professor Marcello Mariani of Reading University, Changjiang Scholar Professor Zhang Yuejun of Hunan University, and Changjiang Scholar Professor Xu Zeshui of Sichuan University.
2. Proposing a theory and methodology for predicting tourism demand based on multiple heterogeneous data sources.
Various data sources have emerged to assist in predicting tourism demand with the development of information technology. Therefore, it is a topic of both theoretical significance and practical value to accurately predict tourism demand based on multiple data sources. To this end, the team has conducted early and systematic research on tourism demand prediction in the context of big data, proposing a series of deep learning prediction methods, including those based on multiple data sources, integrated deep learning prediction, and tourism demand time series data image encoding. The team's proposed theories and methods can effectively improve the accuracy of tourism demand forecasting, providing a scientific basis for government and tourism-related enterprises to make strategic development, investment decisions, revenue management, pricing strategies, and more. Dr. Bi Jianwu has published 8 papers as the first or corresponding author in top international journals in tourism, such as Annals of Tourism Research and Journal of Travel Research. These representative research results have been directly cited and positively evaluated by prominent scholars, such as Professor Nicolau, the Deputy Editor-in-Chief of ATR from Virginia Tech, Professor Yang from Temple University, and academician Wang Shouyang from developing countries.
3. Proposing a method for ranking and recommending tourism products based on online reviews.
More and more people purchase or book products through the internet with the arrival of the Web 2.0 era. Unlike shopping in physical stores, consumers usually cannot experience the products beforehand. They often rely on reading online reviews to gain insights about the product, but with the abundance of online reviews, it can be difficult for consumers to read through them all and make informed decisions. Determining the ranking of products based on online reviews is of great significance in supporting consumer decision-making. The team conducted early and systematic research on ranking and recommending tourism products based on online reviews and proposed a series of ranking and recommendation methods that are important for reducing search time for consumers and promoting purchases. Dr. Bi Jianwu has published 8 papers as the first author or corresponding author, with over 500 citations, in the research area of the online review-based tourism product ranking and recommendation method. Representative research results have been published in international journals such as Annals of Tourism Research, Information Fusion, Information Sciences, and Expert Systems with Applications, and have been directly cited and positively evaluated by Professor Pedrycz, a member of the Canadian Academy of Sciences and editor-in-chief of the international journal Information Sciences, Professor Herrera, editor-in-chief of the international journal Information Fusion from the University of Granada in Spain, Professor Fujita, the former editor-in-chief of the international journal Knowledge-Based Systems from Iwate University in Japan, and Professor Tang from Iowa State University in the United States.
As a pioneer in China's tourism management discipline, the College of Tourism and Service Management has actively shouldered the heavy responsibility of developing the tourism management discipline since its inception and pursued the core proposition of development in the new era. The college has responded to the national major scientific research demands and technological development trends in the management and decision-making research driven by big data, in line with the development of the national strategy of the digital economy, and has deepened the research on tourism big data analysis and intelligent decision-making, promoting the intelligent, digital, and informational development of the tourism industry.
Here are some recent representative achievements:
[1] Bi, J. W, 2023, "Service Attribute Classification and Service Element Allocation: An Approach Based on Sentiment Analysis of Online Reviews", China Social Sciences Press (Funded by the National Social Science Foundation, the monograph was officially published in April 2023)
[2] Bi, J. W., Liu, Y., Fan, Z. P., & Zhang, J. (2019). Wisdom of crowds: Conducting importance-performance analysis (IPA) through online reviews. Tourism Management, 70, 460-478. (ESI highly cited papers)
[3] Bi, J. W., Liu, Y., & Li, H. (2020). Daily tourism volume forecasting for tourist attractions. Annals of Tourism Research, 83, 102923.
[4] Bi, J. W., Li C., Xu H. & Li, H. (2022). Forecasting daily tourism demand with big data: An ensemble deep learning method. Journal of Travel Research, 61(8), 1719-1737.
[5] Bi, J. W., Liu, Y., Fan, Z. P., & Zhang, J. (2020). Exploring asymmetric effects of attribute performance on customer satisfaction in the hotel industry. Tourism management, 77, 104006. (ESI highly cited papers)
[6] Bi, J. W., Li, H., & Fan, Z. P. (2021). Tourism demand forecasting with time series imaging: A deep learning model. Annals of Tourism Research, 90, 103255.
[7] Gao, G. X., & Bi, J. W.* (2021). Hotel booking through online travel agency: Optimal Stackelberg strategies under customer-centric payment service. Annals of Tourism Research, 86, 103074.
[8] Bi, J. W., Liu, Y., Fan, Z. P., & Cambria, E. (2019). Modeling customer satisfaction from online reviews using ensemble neural network and effect-based Kano model. International Journal of Production Research, 57(22), 7068-7088.
[9] Chen, D., & Bi, J.W.* (2022). Cue congruence effects of attribute performance and hosts’ service quality attributes on room sales on peer-to-peer accommodation platforms. International Journal of Contemporary Hospitality Management, 34(10), 3634-3654.
[10] Bi, J. W., Han, T. Y., & Yao, Y. (2023). Fine-grained tourism demand forecasting: A decomposition ensemble deep learning model. Tourism Economics.
[11] Bi, J. W., Han, T. Y., & Yao, Y. (2023). Collaborative forecasting of tourism demand for multiple tourist attractions with spatial dependence: A combined deep learning model. Tourism Economics.
[12] Bi, J. W., Liu, Y., & Fan, Z. P. (2019). Representing sentiment analysis results of online reviews using interval type-2 fuzzy numbers and its application to product ranking. Information Sciences, 504, 293-307.
[13] Bi, J. W., Liu, Y., & Fan, Z. P. (2020). Crowd intelligence: Conducting asymmetric impact-performance analysis based on online reviews. IEEE Intelligent Systems, 35(2), 92-98.
[14] Liu, Y., Bi, J. W.*, & Fan, Z. P. (2017). Multi-class sentiment classification: The experimental comparisons of feature selection and machine learning algorithms. Expert Systems with Applications, 80, 323-339.
[15] Bi, J.W., Han, T. Y., Yao, Y., & Li, H. (2022). Ranking hotels through multi-dimensional hotel information: a method considering travelers’ preferences and expectations. Information Technology & Tourism, 24(1), 127-155.
[16] Bi, J. W., Han, T. Y., & Li, H. (2020). International tourism demand forecasting with machine learning models: The power of the number of lagged inputs. Tourism Economics, 28(3), 621-645.
[17] Cheng, H., Liu, Q., & Bi, J. W.* (2021). Perceived crowding and festival experience: The moderating effect of visitor-to-visitor interaction. Tourism Management Perspectives, 40, 100888.
[18] Liu, Y., Bi, J. W., & Fan, Z. P. (2017). Ranking products through online reviews: A method based on sentiment analysis technique and intuitionistic fuzzy set theory. Information Fusion, 36, 149-161. (ESI highly cited papers)
[19] Liu, Y., Bi, J. W., & Fan, Z. P. (2017). A method for multi-class sentiment classification based on an improved one-vs-one (OVO) strategy and the support vector machine (SVM) algorithm. Information Sciences, 394, 38-52.
[20] Liu, Y., Bi, J. W.*, & Fan, Z. P. (2017). A method for ranking products through online reviews based on sentiment classification and interval-valued intuitionistic fuzzy TOPSIS. International Journal of Information Technology & Decision Making, 16(06), 1497-1522.
[21] Bi, J. W., Liu, Y., & Fan, Z. P. (2020). A deep neural networks based recommendation algorithm using user and item basic data. International Journal of Machine Learning and Cybernetics, 11(4), 763-777.
[22] He, L. Y., Li, H.*,Bi, J.W., Yang, J. J., & Zhou, Q. (2022). The impact of public health emergencies on hotel demand-Estimation from a new foresight perspective on the COVID-19. Annals of Tourism Research, 94, 103402.
[23] Chang, J. L., Li, H.*, &Bi, J.W. (2022). Personalized travel recommendation: a hybrid method with collaborative filtering and social network analysis. Current Issues in Tourism, 25(14), 2338-2356.
[24] Bi, J.W, Liu, Y. Fan, Z. (2018). Method for ranking products through online reviews. Journal of Systems Engineering, 33(03):422-432.
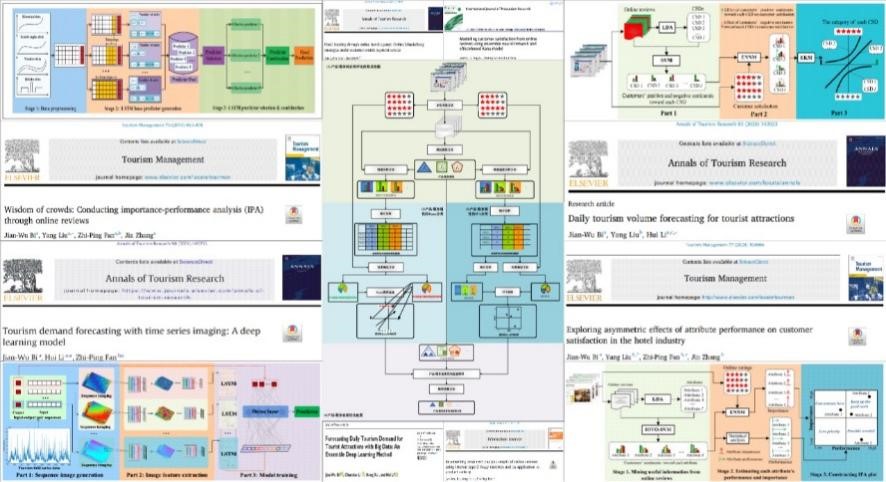
(Translator:BI Jianwu,Proofreading:LI Chunxiao)